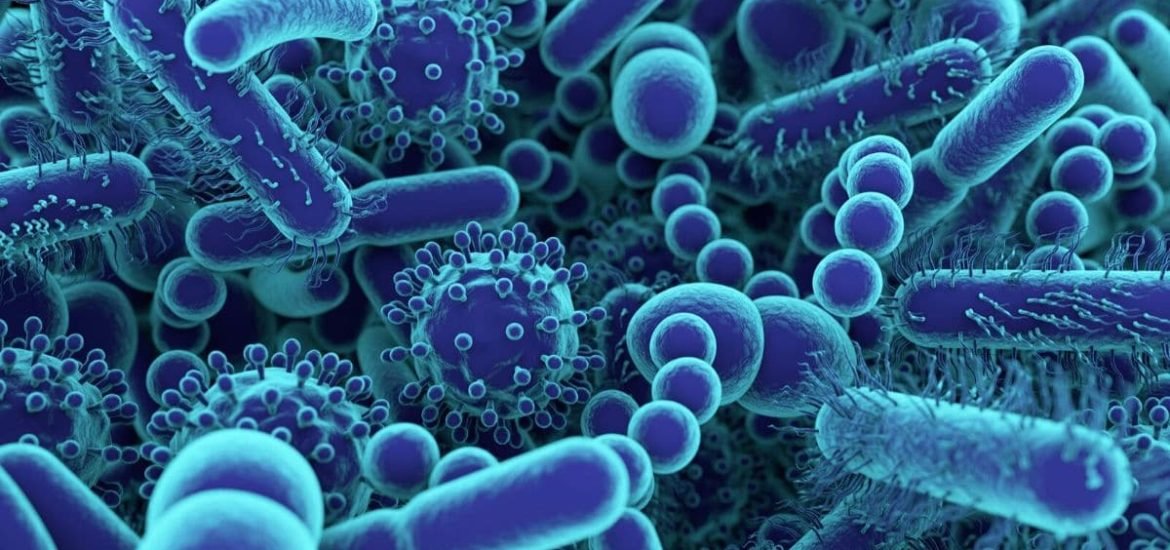
Scientists are using machine learning to map microbial communities in the human body. Two new papers published this week in PLOS One and Science provide some incredible insights into the function of specific microbes in both healthy and diseased humans.
The microbiome as an important indicator of health
Groups of bacteria and viruses naturally coexist throughout the human body. Over the past few decades, scientists have made tremendous headway in understanding the influence of the so-called human microbiome on digestion, metabolism, and fighting disease – from inflammatory bowel disease to diabetes, obesity, cancer, and even depression.
More precisely, imbalances in the microbiome might be the source of many health problems. Indeed, the human microbiome is increasingly recognised as an indicator of health and disease, and specific links can be identified between certain microbe communities and health.
However, the complexity of the microbial ecosystem — there are thousands of different species of microbe in the human body — makes it difficult to identify the contribution of individual microbial communities to health and disease. Moreover, communities of bacteria, viruses, and fungi vary from tissue to tissue and also differ among individuals. Therefore, correcting potentially disease-causing microbial imbalances remains a challenge. So, researchers are turning to artificial intelligence in the hopes of gleaning some answers.
Machine learning is an invaluable tool in biology
A massive amount of genetic sequencing data has become available in the past few years, providing a treasure trove of information. This has led to an entirely new field of research known as metagenomics. By using advanced computational methods, scientists piece together genomic data from biology to tell the stories of biology. For example, researchers previously used machine learning to decipher links between age and the microbiome. In another study, a similar approach provided clues about ancient evolution.
Coupled with the abundant data being shared through public databases, artificial intelligence is an incredibly useful tool in biology. Machine learning can uncover new insights into the complex workings of the human body and might even contribute to developing new and better drugs to treat diseases, including those linked to deviations in the microbiome.
Understanding the complex microbial ecosystem
To better understand how microbial communities perform crucial functions within the complex microbial ecosystem, researchers at the University of Drexel used a combination of high-throughput genetic sequencing and language-processing algorithms to decode human microbial communities and reveal how they operate, as the team reports on 11 December in PLOS One (1).
The scientists are seeking to answer important questions like: What do microbial communities look like? How prevalent they are? How their internal configuration affect their immediate environment within the human body?
But as co-author Dr Gail Rosen, an associate professor of engineering at Dexel, said: “We are really just beginning to scrape the surface of understanding the health effects of microbiota”.
The team uses machine learning algorithms to spot recurring patterns in genetic code — DNA and RNA — that indicate which microbes are often found together. They have coined the method ‘themetagenomics’, since they are looking for recurring themes in microbiomes, which will help inform the extent of the community and how frequently and where else they might be occurring.
In this way, they hope to reveal what each microbe community is doing. “It’s hard to develop treatments to promote natural microbial coexistence if their ‘natural state’ is not yet known”, says co-author Dr Steve Woloszynek.
Then, by observing which microbes co-exist and how these communities change over time in both healthy and diseased individuals, the researchers hope to use the presence of certain microbe as indicators of diseases like Crohn’s or even specific types of cancer. And perhaps, even develop targeted treatments.
‘Chemical conversations’ of the microbiome
In a nearby lab at Princeton Univesity, Sugimoto and colleagues use similar methods to listen to ‘chemical conversations’ between various microbes of the human microbiome. A new paper published on 13 December in Science explores some of these chemical cues (2).
The researchers first set out to identify the sets of genes called biosynthetic gene clusters, or BGCs, that are involved in producing certain chemicals. Then, they were able to uncover which groups of microbes use these particular chemical signals to communicate. But this would not have been achieved without the help of computer algorithms.
Once Donia and his team had identified the genes essential for manufacturing a particular chemical of interest, computer algorithms were used to look for similar genetic sequences among the so-called metagenomic data from tissues of hundreds of people.
“Our long-term goal is to define the chemical space of the human microbiome,” explained Dr Mohamed Donia, an assistant professor of Molecular Biology at Princeton.
To their surprise, they discovered 13 BGCs widely distributed in the gut, oral, and skin microbiome. Incredibly these gene clusters were present in diverse populations around the world — ”from the US to Fiji”. Then, when the researchers purified and tested two of those BCGs for antibiotic or anticancer activity, they found that two out of the five new molecules produced by the gene clusters were potent antibiotics.
The discovery of novel antibiotics and other biological compounds has become immensely important as more and more pathogens evolve resistance to the antibiotics currently in clinical use.
(1) Woloszynek, S. et al. PLOS ONE (2019). Exploring thematic structure and predicted functionality of 16S rRNA amplicon data. DOI: 10.1371/journal.pone.0219235
(2) Sugimoto, Y. et al. A metagenomic strategy for harnessing the chemical repertoire of the human microbiome. Science (2019). DOI: 10.1126/science.aax9176