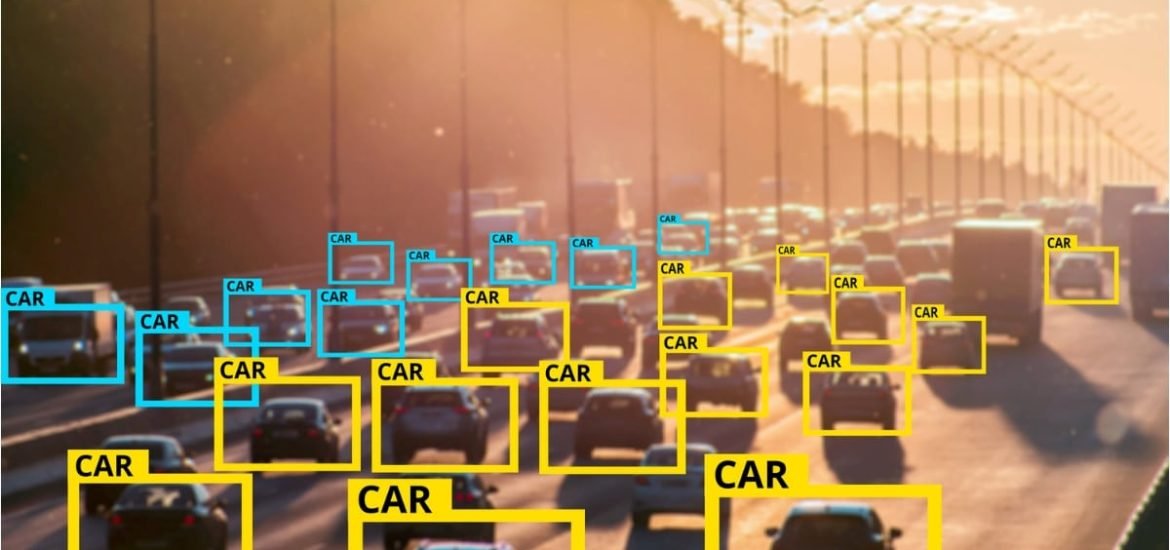
Machine learning algorithms are designed to mimic neural networks of the human brain, but can the human brain think like a computer? According to a new study that appeared on 22 March in Nature Communications, it can (1). The findings suggest that human intuition can provide new insights into how machines classify images, even those images designed to fool machines.
Artificial intelligence can classify images with remarkable accuracy, at rates approaching those of adult humans. However, there is one big problem, these Convolutional Neural Networks (CNNs) remain vulnerable “fooling” images and “perturbed” images ― images are intentionally created so that neural networks cannot correctly identify them. This blind spot could be exploited by hackers to carry out malicious attacks.
Such adversarial attacks that seek to “fool” computers, or even modified objects that are simply unrecognisable to computers, greatly diminish the usefulness of image-recognition systems, and present a serious stumbling block for autonomous systems like self-driving cars, as well as facial recognition software and radiological diagnostics.
Fooling images suggest that humans and machines see images differently, which can lead to machines misidentifying objects in ways that humans would not. Whereas humans have the ability to decide whether an object just looks like something or is actually that thing ― for example, a cloud that looks like an animal ― neural networks can’t make the same distinction. But does that mean humans can’t see the images the same way computers do?
To shed some light on the “thought process” of computers, instead of trying to get computers to think like people, the researchers wanted to find out whether humans can think like machines.
A total of 1800 volunteers participated in the experiments. The volunteers were presented with dozens of fooling images that had previously tricked computers and provided with two labelling options ― the computer’s answer and another randomly generated option. They were asked to “think like a machine” and determine which label they thought was generated for each image by the image recognition system. Incredibly, the researchers found that people and computers chose the same answer 75 per cent of the time.
Then to make things even harder, the participants were offered a choice between the computer’s favourite answer and its next-best guess. And 91 per cent of the human participants agreed with the machine’s first choice.
The results suggest that when humans are placed in the same scenarios and told to think like a computer, they tend to agree with the machine. So, what does this mean?
The authors write, “Human intuition may be a surprisingly reliable guide to machine (mis)classification—with consequences for minds and machines alike.” In other words, the fooling images that trick machines can also trick humans under the same conditions. But if humans can see the ways a computer recognition system can be tripped up by fooling images, this could present a potential defence against misclassification.
(1) Zhou, Z. and Firestone, C. Humans can decipher adversarial images. Nature Communications (2019). DOI: 10.1038/s41467-019-08931-6