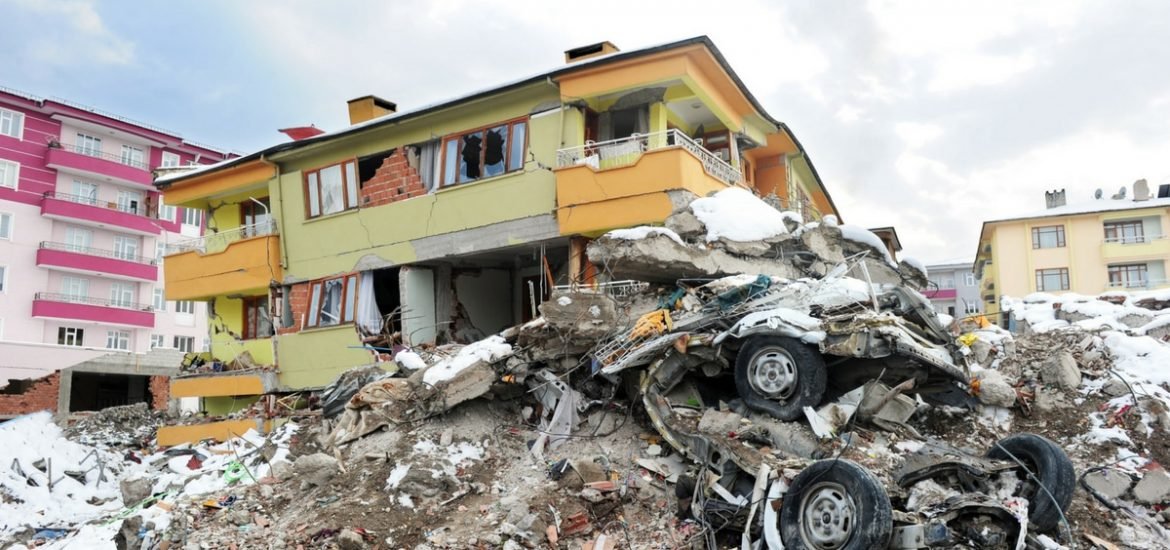
A new model based on machine learning can predict the location and timing of earthquake aftershocks with more precision than the standard method, known as the Coulomb failure criterion. The study published in Nature on 29 August used neural networks to predict where and when earthquake aftershocks would occur (1). The study was carried out by Prof Phoebe DeVries, a seismologist at Harvard University in Cambridge, Massachusetts, and her team. Devries told Scientific American, “We’ve really just scratched the surface of what machine learning may be able to do for aftershock forecasting.”
Aftershocks can be just as devastating as the main earthquake and continue for months or even years after the main event. Aftershocks of the 2011 Japan earthquake were felt for more than 5 years. In 2010, a magnitude-7.1 earthquake near Christchurch, New Zealand, did not result in any fatalities but the magnitude-6.3 aftershock more than 5 months later was much closer to the city centre and caused 185 deaths. Aftershocks are usually clustered and seismic activity tends to decay over time. But fault orientations in the subsurface are extremely complicated making it difficult to predict where and when these subsequent events will occur.
Artificial neural networks ― inspired by the neural network of the human brain ― are widely used in machine learning. They are behind some of the biggest advances in artificial intelligence over the past decade ― from self-driving cars to detecting ‘fake news’ ― because they are excellent at finding patterns too complex for regular computer programs to extract. Neural networks are made up of input and output layers, as well as hidden layers. These hidden layers are somewhat of a “black box” and look at numerous potential paths in order to transform the input into something usable, such as a probability or prediction.
The neural network used in this study ― consisting of six hidden layers with 50 neurons each ― was trained using data on more than 131,000 earthquakes and their aftershocks. The data included both the locations and magnitudes, as well as changes in stress on the faults. Learning from this data, neural networks could actually pin down aftershock locations using data from an independent test set containing another 30,000 earthquake-aftershock pairs and were able to determine the probability of an aftershock event occurring at a given location. The analysis consistently predicted aftershock locations much better than the Coulomb failure criterion.
The findings are a new take on aftershock triggering that will be helpful in understanding the mechanisms behind these potentially catastrophic events. According to the authors, “this machine-learning-driven insight provides improved forecasts of aftershock locations and identifies physical quantities that may control earthquake triggering during the most active part of the seismic cycle.” In other words, neural networks could be used to develop new methods for assessing aftershock risks during the subsequent― and most high-risk― days and weeks, with a view to preventing them or limiting their effects and potentially saving lives.
(1) DeVries, P. M. R. et al. Deep learning of aftershock patterns following large earthquakes (2018). DOI: https://doi.org/10.1038/s41586-018-0438-y
Image source: Getty Images