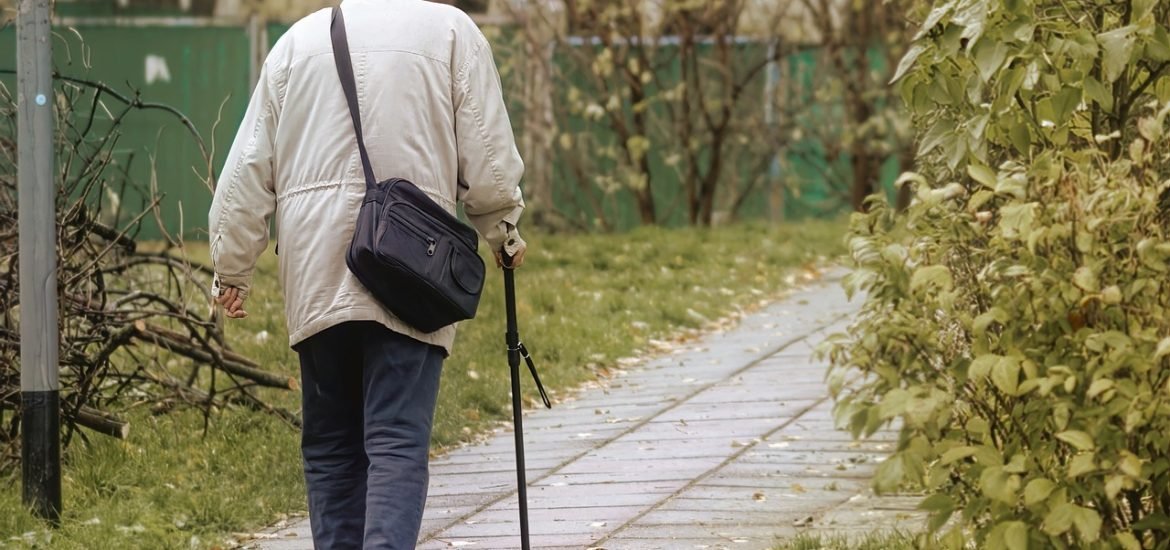
Video-enabled glasses can help patients at risk of falls by allowing medical staff to monitor their movements, but this new technology has many privacy concerns. A team of scientists from Northumbria University, UK, discuss this issue and assess whether AI could be used to ensure patient privacy. This work was published in Nature Digital Medicine.
Until now, patients at risk of falls have frequent appointments with their doctors to discuss potential problems. However, this relies on information the patient provides and is not very objective. Wearable technology worn on the lower back can track the patient’s movements and posture, but the information gathered is still limited and out of context. For example, it doesn’t identify what the patient is carrying, for example.
“As you can imagine, the variability in how people move when they’re completing different tasks is enormous,” said Dr Alan Godfrey, Associate Professor in Computer and Information Sciences at Northumbria University. “The data or information provided from an inertial wearable device attached to someone when they’re wandering around their house will inevitably differ from the same person when they’re outdoors or walking more quickly to get somewhere or to keep up with someone. This means that while the use of an inertial wearable alone is helpful, it tells us nothing about the context in which a fall may occur. Having absolute clarity about the environment and what people are doing is key.”
In a bid to improve patient care, experts have been exploring how they can capture better data about the patient’s gait and posture with wearable devices. One option is to ask patients to wear video-enabled glasses. These devices provide more accurate information on how patients move around and how they deal with obstacles and other hazards. The problem is finding a way to ensure that patients always retain their privacy.
To find a solution, a group of computer experts “trained” a new AI software to blur personal data and information captured by video glasses, such as footage of children or confidential documents. Combining this with wearable device recording gait data allowed clinicians to have a more comprehensive picture of how patients move in their own surroundings. This method significantly improves how doctors assess patients at risk of falling and helps in the decision-making process around patient care.
“Traditionally the use of video within the home has caused some apprehension among patient populations as a result of privacy concerns owing to what else may be captured on camera. However, through the use of AI software that can identify and obscure personal or sensitive information, we can effectively capture the contextual information that will allow us to better understand abnormal gait data whilst overcoming the concerns patients may have around the use of video technology in their own homes,” said PhD student Jason Moore, from the Department of Computer and Information Sciences at Northumbria University. “The benefit of providing this contextual information is that clinicians will have a fuller picture for each individual patient, which could ultimately allow them to provide more informed care plans and potentially keep more patients in their own homes for longer.”
Dr Godfrey continued: “The suggested application of this technology is unique. The way it works means that the raw footage would never be seen by clinicians, however the contextual information it could provide when it comes to identifying potential reasoning for abnormal variability or asymmetry in gait – effectively the way patients carry themselves and move around which are signs of elevated fall risk – is invaluable.
“Without this context, patients with numerous clinical conditions, for example, Parkinson’s, those who’ve suffered strokes, or even just those who are suffering from frailty, could find themselves being classed as high risk for falls and ultimately face difficult decisions around moving out of their own environment earlier than necessary. It also alleviates the pressures on patients to travel to bespoke clinics for monitoring, replacing this with a community-based approach. It could go a long way to help us truly understand the fall risk of a patient and to provide personalised care solutions that are most appropriate to the individual and their needs.”
Moore, J., McMeekin, P., Parkes, T. et al. Contextualizing remote fall risk: Video data capture and implementing ethical AI. npj Digit. Med. 7, 61 (2024). https://doi.org/10.1038/s41746-024-01050-7