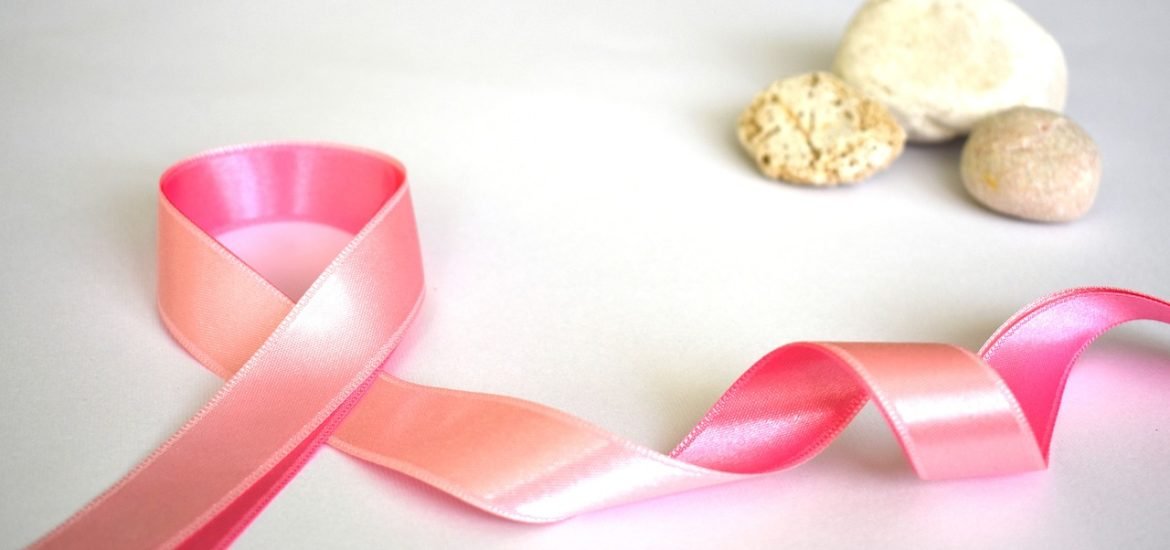
Women may have access to better treatment for breast cancer as new AI technology enables earlier detection of damaged cells, according to a study published in The Lancet Digital Health. The authors from the University of Copenhagen suggest that the new system is better at predicting the risk of developing breast cancer than current methods.
Breast cancer is one of the most common forms of cancer. To help patients, a team of researchers from the University of Copenhagen uses AI to scan for irregular-looking cells to give women a better risk assessment and predict possible breast cancer cases. The authors defend that AI technology is far better at predicting cancer risk than current clinical benchmarks for risk assessment.
In this study, the team used deep learning AI to analyse mammary tissue samples from donors to look for signs of damaged cells, an early indicator of cancer. “The algorithm is a great leap forward in our ability to identify these cells. Millions of biopsies are taken every year, and this technology can help us better identify risks and give women better treatment,” said Associate Professor Morten Scheibye-Knudsen from the Department of Cellular and Molecular Medicine.
A crucial aspect of assessing cancer risk is identifying dying cells that are still metabolically active but have stopped dividing. These cells can cause inflammation and lead to cancer. “We sometimes refer to them as zombie cells because they have lost some of their function, but they are not quite dead. They are associated with cancer development, so we developed and trained the algorithm to predict cell senescence. Specifically, our algorithm looks at how the cell nuclei are shaped because the nuclei become more irregular when the cells are senescent,” explained Indra Heckenbach, first author of the study.
AI allowed the team to spot these cells in tissue biopsies and predict the risk of breast cancer better than the Gail model, the current gold standard for assessing breast cancer risk. “We also found that if we combine two of our own models or one of our models with the Gail score, we get results that are far better at predicting the risk of getting cancer. One model combination gave us an odds ratio of 4.70, which is huge. It is significant if we can look at cells from an otherwise healthy biopsy sample and predict that the donor has almost five times the risk of developing cancer several years later,” said Indra Heckenbach, first author of the study.
The technology is not ready for patients, but it should be relatively easy to implement because the system only needs tissue images. “We will be able use this information to stratify patients by risk and improve treatment and screening protocols. Doctors can keep a closer eye on high-risk individuals, they can undergo more frequent mammograms and biopsies, and we can potentially catch cancer earlier. At the same time, we can reduce the burden for low-risk individuals, e.g., by taking biopsies less frequently,” concluded Morten Scheibye-Knudsen.
Heckenback I, Powell M, Fuller M eat al (2024) Deep learning assessment of senescence-associated nuclear morphologies in mammary tissue from healthy female donors to predict future risk of breast cancer: a retrospective cohort study. The Lancet Digital Health, Volume 6, e681 – e690