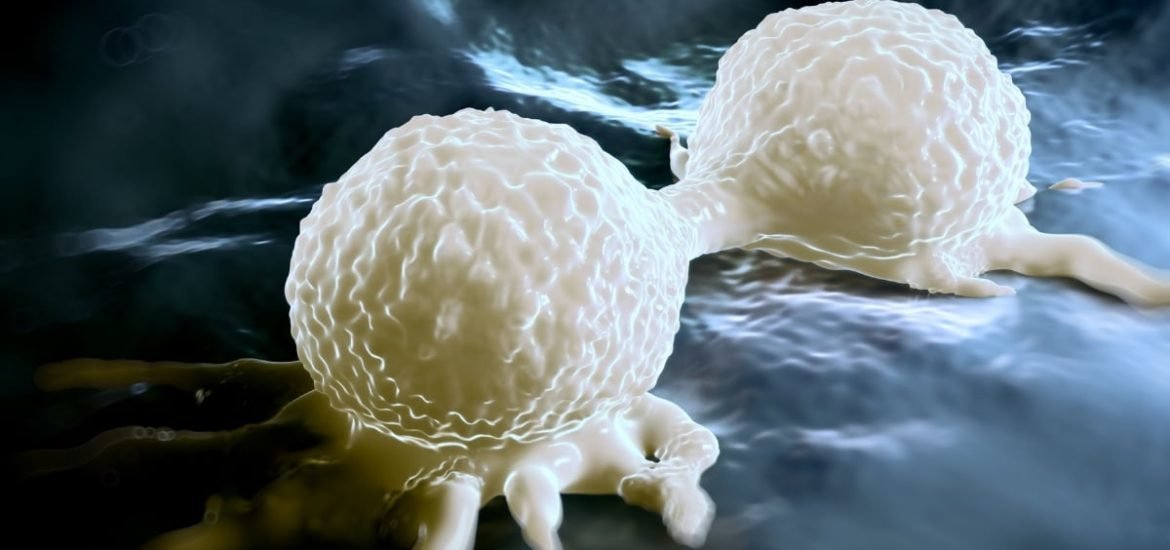
In a new study, published on 10 September in Nature, a scientific journal, researchers from the European Molecular Biology Laboratory (EMBL), Germany and the Research Institute of Molecular Pathology, Austria integrated CRISPR/Cas9 technology, confocal microscopy, and machine learning to investigate the dynamics of specific proteins involved in mitosis. According to the authors, they have provided, “an integrated experimental and computational framework to build a comprehensive and quantitative 4D model of the mitotic protein localization network in a dividing human cell.”
Mitosis is the process by which one cell divides to create two identical cells ― one of the most fundamental processes for life ― and is important for growth and also to replace damaged or injured cells. Previously, in 2010, the same group were able to identify parts of the human genome required for human cell division, as part of the EU MitoCheck project. However, the actual cell division process is carried out by proteins encoded by these genes and requires the close coordination of hundreds of proteins working in specialized groups.
In this study, researchers used CRISPR/Cas genome editing to fluorescently label 28 mitosis-related proteins in HeLa cells, a widely used cancer cells line. Then, three-dimensional (3D) confocal microscopy was used to track the proteins and uncover where they are located (and how many of them) and at certain time points during the cell division process. Finally, the data was integrated into an interactive computer model ― based on machine learning which was used to train a regression model to assign the amount of protein to different reference compartments, allowing the flow of proteins to be quantitatively compared.
The resulting Mitotic Cell Atlas can be used by scientists to observe protein interactions and where proteins are located in real time during cell division. According to the paper, this research “can be applied to systematically map and mine dynamic protein localization networks that drive cell division in different cell types, and can be conceptually transferred to other cellular functions.” Exploring and mining data within the atlas can be used to develop new hypotheses on the various functions of cellular proteins and the modelling framework could be adapted to study other vital biological functions, such as cell migration and differentiation.
Furthermore, cellular processes can go wrong, leading to diseases like cancer, and examining disease-relevant processes in this way may uncover important information that could be used to treat or prevent disease. Therefore, the experimental methods, microscopy techniques, and code used to create the dynamic protein atlases have been made open-source to allow further progress in this area.
Using the pilot dataset, the authors have thus far demonstrated the power of their model “by mining the data to automatically define dynamic localization patterns to subcellular structures as well as predicting the order, stoichiometry and rates of assembly and disassembly of subcellular organelles.” They also suggest “this quantitative information on protein localization in living cells provides greater insights into protein dynamics and interactions at relevant temporal resolutions and supports simulations of mitotic processes.”
Completing the dataset, which would include around 600 different proteins, will take several more years but will enable researchers to better understand the mechanisms involved in mitosis.
(1) Cai, Y. et al. Experimental and computational framework for a dynamic protein atlas of human cell division. Nature (2018). DOI: 10.1038/s41586-018-0518-z