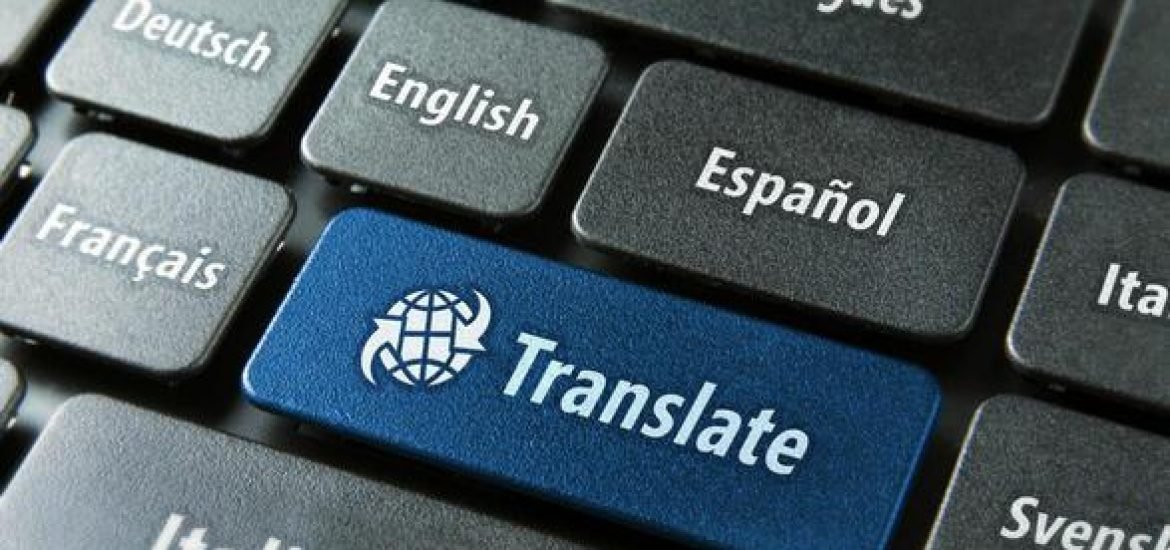
Two papers offer up a novel method showed some artificial intelligence programs can each themselves new language – without any dictionary or human help.
In this piece, we will not discuss Google translation’s tedious gibberish. The program even was discarded by the company last year, and was replaced by a highly efficient neural network able to translate whole sentences, taking their context into account thanks to supervised automated learning. However, recently two artificial intelligence programs (AI) have recently successfully translated whole bodies of text without relying on an integrated dictionary.
Two teams of researchers – one French, one Spanish – working independently on two separate projects, with no connection of even knowledge of the other team’s endeavour, managed to create programs able to learn languages on their own. To do so, they used the significant multilingual databases provided by the European Parliament and the UN.
The first study was led by Mikel Artetxe, a computer scientist at the University of the Basque Country (UPV). The second one was supervised by Guillaume Lample, a French engineer working for Facebook’s artificial intelligence department. Both teams have created unsupervised machine learning methodology based on word analogy that enable their AIs to translate. Both of them rely on a quite similar approach.
“Imagine giving loads of different Chinese and Arabic books to someone”, Mikel Artetxe explained. “This person now has to learn to learn to translate into both languages without any cross referencing.” To understand the potential of these new systems, it helps to know how current machine translation works. Ahuman-supervised neural network compares books and articles that have been previously translated by humans. By comparing extremely large amounts of these parallel texts, they can learn equivalences between any two given languages.
The new systems, build a map of all the connections they find between the words of the languages they are trying to translate. The technique is the same as for a digital road atlas. Since languages group words in similar ways, the systems guess what the word equivalencies are, building translation dictionaries with that information.
One of the two systems checks the final result by retranslating the sentence back into the source language – and compares it to the source material to correct its work. The other one adds or takes words away to the source material and compares results. So far, however, both IA’s performances still can be improved – and still pale when compared with human work. Nevertheless, the two new papers demonstrate that it’s possible to develop a system that doesn’t rely on parallel texts.
This post is also available in: FR (FR)DE (DE)